The automotive industry continues to be a hotbed of patent innovation. The automotive industry is leveraging the increasing availability of data to develop end-to-end learning models for tasks such as driving and object detection. The affordability of computing has made it more feasible to use these models in real-time applications like autonomous driving. The growing demand for autonomous vehicles also makes end-to-end learning models a promising approach for developing autonomous vehicle software. The automotive industry is utilizing deep learning, generative adversarial networks (GANs), reinforcement learning, and simulation-based training for end-to-end learning models, enhancing tasks such as image recognition, natural language processing, and machine translation, while minimizing damage to vehicles or people. In the last three years alone, there have been over 720,000 patents filed and granted in the automotive industry, according to GlobalData’s report on Artificial intelligence in automotive: end-to-end learning models. Buy the report here.
However, not all innovations are equal and nor do they follow a constant upward trend. Instead, their evolution takes the form of an S-shaped curve that reflects their typical lifecycle from early emergence to accelerating adoption, before finally stabilizing and reaching maturity.
Identifying where a particular innovation is on this journey, especially those that are in the emerging and accelerating stages, is essential for understanding their current level of adoption and the likely future trajectory and impact they will have.
300+ innovations will shape the automotive industry
According to GlobalData’s Technology Foresights, which plots the S-curve for the automotive industry using innovation intensity models built on over one million patents, there are 300+ innovation areas that will shape the future of the industry.
Within the emerging innovation stage, self-driving neural networks, autonomous lane changing and AV simulation and testing are disruptive technologies that are in the early stages of application and should be tracked closely. Predictive acceleration control, collaborative autonomous driving, and AV perception and control systems are some of the accelerating innovation areas, where adoption has been steadily increasing.
Innovation S-curve for artificial intelligence in the automotive industry
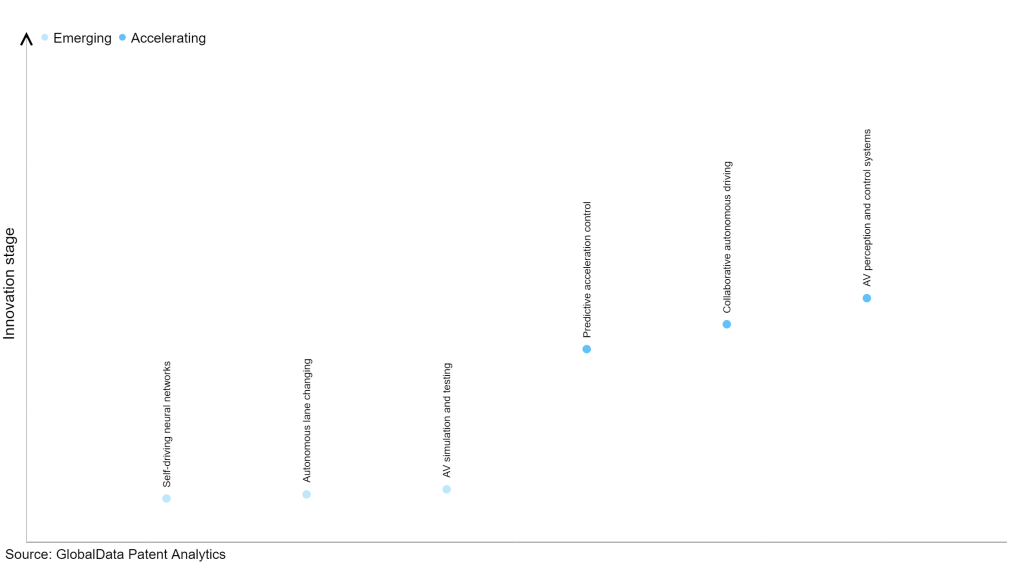
End-to-end learning models is a key innovation area in artificial intelligence
End-to-end learning models refer to machine learning models that take raw input data and directly output an action or decision without the need for manual feature engineering or intermediate steps. These models learn from the raw data to make decisions or actions.
GlobalData’s analysis also uncovers the companies at the forefront of each innovation area and assesses the potential reach and impact of their patenting activity across different applications and geographies. According to GlobalData, there are 540+ companies, spanning technology vendors, established automotive companies, and up-and-coming start-ups engaged in the development and application of end-to-end learning models.
Key players in end-to-end learning models – a disruptive innovation in the automotive industry
‘Application diversity’ measures the number of applications identified for each patent. It broadly splits companies into either ‘niche’ or ‘diversified’ innovators.
‘Geographic reach’ refers to the number of countries each patent is registered in. It reflects the breadth of geographic application intended, ranging from ‘global’ to ‘local’.
Patent volumes related to end-to-end learning models
Source: GlobalData Patent Analytics
Capital One Financial, the top patent filer in automotive end-to-end learning models. One of the patents related to the technology describes techniques for monitoring application performance and dependencies involve a monitoring application that collects and reports statuses using existing interfaces, without altering the monitored application or its dependencies. It identifies root causes of issues and uses dependency analyzers and discovery crawlers for automatic configuration. Machine learning analyzes performance patterns and provides health reports against baseline statuses. Additionally, testing the monitored application’s response via modified API calls helps train the machine learning model. Smith & Nephew, Microsoft, and Intel are some of the other leading patent filers in end-to-end learning models.
In terms of application diversity, Smith & Nephew leads the pack. Microsoft and Tractable stood in the second and third positions respectively. By means of geographic reach, Smith & Nephew held the top position followed by Tractable and FiveAI.
To further understand the key themes and technologies disrupting the automotive industry, access GlobalData’s latest thematic research report on Artificial Intelligence (AI) in Automotive.
Data Insights
From
The gold standard of business intelligence.
Blending expert knowledge with cutting-edge technology, GlobalData’s unrivalled proprietary data will enable you to decode what’s happening in your market. You can make better informed decisions and gain a future-proof advantage over your competitors.